A diffractive neural network that can be flexibly programmed
[ad_1]
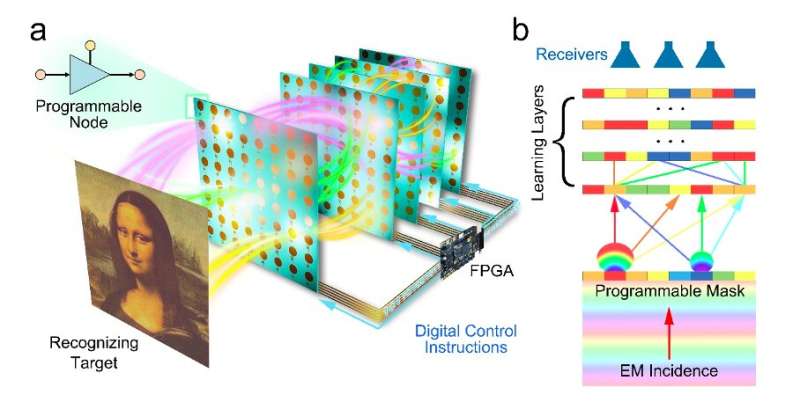
In recent decades, machine learning and deep learning algorithms have become increasingly advanced, so much so that they are now being introduced in a variety of real-world settings. In recent years, some computer scientists and electronics engineers have been exploring the development of an alternative type of artificial intelligence (AI) tools, known as diffractive optical neural networks.
Diffractive optical neural networks are deep neural networks based on diffractive optical technology (i.e., lenses or other components that can alter the phase of light propagating through them). While these networks have been found to achieve ultra-fast computing speeds and high energy efficiencies, typically they are very difficult to program and adapt to different use cases.
Researchers at Southeast University, Peking University and Pazhou Laboratory in China have recently developed a diffractive deep neural network that can be easily programmed to complete different tasks. Their network, introduced in a paper published in Nature Electronics, is based on a flexible and multi-layer metasurface array.
“Our hope was to realize a diffractive neural network in which each unit can be independently and flexibly programmed,” Tie Jun Cui, one of the researchers who carried out the study, told TechXplore. “Drawing inspiration from our past research on digital programmable metasurfaces, information metasurfaces and experience in electromagnetic regulation, we created a programmable diffractive neural network machine by constructing multilayer programmable transmissive metasurfaces, which we named programmable artificial intelligence machine (PAIM).”
The researchers’ diffractive neural network performs calculations that closely resemble those performed by optical diffractive neural networks. More specifically, their network performs matrix network operations when electromagnetic waves propagate through its multi-layer metasurfaces, which resemble the light speed calculations performed by optical diffractive networks.
“The key breakthrough of our work is that each neuron is independently reprogrammable,” Cui explained. “Therefore, the entire neural network can be trained and programmed on-site and can also be repeatedly trained according to different task requirements. We design a metasurface unit (neuron) with reprogrammable transmission coefficients that integrates a power amplifier chip.”
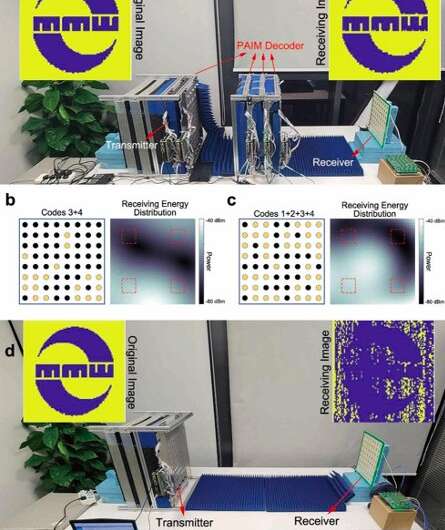
Every amplifier in the researchers’ network can be digitally controlled through a field-programable gate array (FPGA). FPGAs are systems that contain an array of different programmable logic blocks or units. In the system created by Cui and his colleagues, every individual unit can be independently controlled, which allows engineers to program the entire neural network and allow it to perform well in specific tasks.
“The biggest highlight of our study was the realization of a programmable diffractive neural network in a convenient and efficient way,” Cui said. “In the past, optical diffraction deep neural networks were mainly composed of optical media such as silicon dioxide, which were non-adjustable materials. Therefore, this type of optical neural network needs to be trained with the help of a computer, and the final network distribution is obtained before processing.”
Most previously developed diffractive optical neural networks remained fixed once they are trained. As a result, they can only complete a set number of tasks, those they were originally trained on. In contrast, as it is based on digital metasurfaces, the network created by Cui and his colleagues can be programmed to complete different tasks.
“Programmable metasurfaces can control electromagnetic waves with a simple architecture, low cost and high efficiency, which is a potential choice for building programmable neural networks,” Cui added. “Our PAIM can also directly modulate free-space electromagnetic waves with light speed, which is a potential low-latency signal processing unit used for 5G and 6G wireless communications.”
In initial evaluations, the diffractive neural network introduced by this team of researchers achieved very promising results, as it was found to be highly flexible and applicable across a wide range of scenarios. In the future, it could thus be used to solve a variety of real-world problems, including image classification, wave sensing and wireless communication coding/decoding. Meanwhile, Cui and his colleagues will work on improving its performance further.
“The prototype implemented in this work is based on a 5-layer diffractive neural network, each layer has 64 programmable neural networks, and the total number of nodes in the network is relatively low,” Cui added. “At the same time, the operating frequency band of this network is lower, resulting in a larger size of the physical network. In our next studies, we plan to further increase the scale of the programmable neurons of the network, improve the network integration, reduce the size and form a set of intelligent computers with stronger computing power and more practicality for sensing and communications.”
Che Liu et al, A programmable diffractive deep neural network based on a digital-coding metasurface array, Nature Electronics (2022). DOI: 10.1038/s41928-022-00719-9
© 2022 Science X Network
Citation:
A diffractive neural network that can be flexibly programmed (2022, March 24)
retrieved 4 April 2022
from https://techxplore.com/news/2022-03-diffractive-neural-network-flexibly.html
This document is subject to copyright. Apart from any fair dealing for the purpose of private study or research, no
part may be reproduced without the written permission. The content is provided for information purposes only.
[ad_2]
Source link